Intelligence on the Edge
Strategic Research Area 3
Distributed Intelligence
Guided by Associate Professor Miguel Bordallo López, this strategic research area recognises data as the cornerstone of the future digital society. The anticipated massive data streams resulting from the ultra-densification of communications and sensing pose a significant challenge to the existing data and computing infrastructures.
In more detail, the onslaught of generated data calls for distributing computations over the device-edge-cloud computing continuum. Along the continuum, novel distributed paradigms for AI/ML model training and inference are required, leveraging the capabilities of advanced 6G networks to enhance privacy and data locality. Distributed computing and AI/ML will play pivotal roles in enabling distributed sensing, modelling, and the development of unique user interfaces.
These advancements will need integration into complex system architectures that operate independently and in dynamic contexts, thereby laying the solid foundation needed to meet the future society’s demands.
Distributed INTELLIGENCE
Research Themes
Computing on the edge-to-cloud continuum
Massive volumes of locally created data and tightened latency, security, and privacy constraints render the old cloud-native model obsolete. Instead, edge and fog computing bring cloud computing closer to applications, data sources, and regulated processes.
Distributing computation across the edge-to-cloud continuum needs a network of dynamically linked nodes that share resources. This is useful in contexts where data is constantly changing, and latency requirements are high. Edge computing enables optimizing signal-processing operations among cloud, edge servers, radio heads, and devices. Self-aware computing and Information-Centric Networking enable such orchestration.
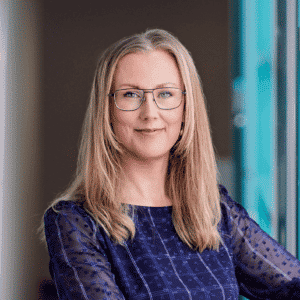
Theme Leader
Susanna Pirttikangas
View bioDistributed AI
Future data-intensive applications need distributed AI and analytics solutions on the edge and fog computing platforms. With the advent of 6G, these solutions will help complement human decision-making, construct autonomous systems from small devices to whole factories, and optimize network performance and marshal billions of devices.
Achieving varied service and latency needs while increasing resource efficiency is the key challenge of future AI. Updated application-level protocol and security solutions are required.
We develop ways to maximize AI method distribution across heterogeneous nodes. Increasing heterogeneous streaming data will demand sophisticated computing paradigms and distributed and self-organizing methods. Furthermore, achieving system-level goals and efficiently employing resources needs system models for decision making.
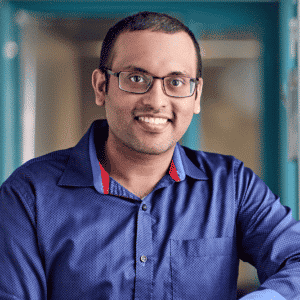
Theme Leader
Sumudu Samarakoon
View bioMultimodal sensing and modelling
6G relies on multimodal sensor data to detect and model the surroundings. New sensors and actuators accompanied with high-speed connectivity and low-cost computational processing have made real-time and distributed intelligent applications feasible.
The challenge is to make sense of all the data. Uncertainty quantification and propagation are automated ways to improve operational data quality and privacy. It is possible to increase the trustworthiness of smart decision support systems by improving data visibility from several sources and understanding the functions and logic behind the judgments.
6G is intended to natively allow radio-based sensing and support ultra-dense sensor and actuator networks, enabling hyper-local and real-time sensing, communication, and interaction. Both the physical and programmed worlds require multidimensional orchestration.
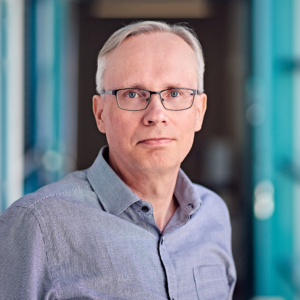
Theme Leader
Janne Heikkilä
View bioDistributed INTELLIGENCE
Publication Highlights
Ding, A. Y., Peltonen, E., Meuser, T., Aral, A., Becker, C., Dustdar, S., … Wolf, L.
Roadmap for edge AI: a Dagstuhl perspective
Elgabli, A., Park, J., Bedi, A. S., Bennis, M., Aggarwal, V.
GADMM: Fast and Communication Efficient Framework for Distributed Machine Learning
Lähderanta, T., Leppänen, T., Ruha, L., Lovén, L., Harjula, E., Ylianttila, M., … Sillanpää, M. J.
Edge computing server placement with capacitated location allocation
Bhayani, S., Sattler, T., Barath, D., Beliansky, P., Heikkilä, J., Kukelova, Z.
Calibrated and Partially Calibrated Semi-Generalized Homographies
Niu, X., Yu, Z., Han, H., Li, X., Shan, S., Zhao, G.
Video-based remote physiological measurement via cross-verified feature disentangling
Distributed Intelligence
Solutions and Development
Our practical solutions consider microservices-based edge architectures which decrease the delays of data-intensive applications while providing security and privacy to the users. The architectures are particularly well suited for environments where data are dynamically changing and latency requirements for data exchange are very stringent.
Read more in the White Paper on 6G Drivers and the United Nations SDGs.
Development
We will also develop new distributed learning mechanisms to allow algorithms to run at edge servers, user terminals and other devices with limited data while providing strong robustness against device and link failures.
SEE THE ACTION
Sample projects
ILLUSIVE: Foundations of Perception Engineering
FRACTAL: Empowering Edge Computing
MAALI: Multisensory automation for assisted living
Frages: A framework for General Spatial AI
R2D2: Robust and Reliable Delay-sensitive Communication and Control Co-design
Contact us to learn more on
Distributed Intelligence Research?
To learn more about distributed intelligence research, contact a theme leader directly or drop a message to our SRA coordinator.
Check out our other strategic research areas
6G Flagship research seeks scientific 6G breakthroughs in four interconnected strategic research areas. Learn more about our other strategic research areas.
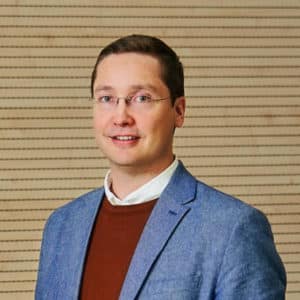
Strategic Research Area Coordinator